Content-based search for deep generative models
Daohan Lu | Sheng-Yu Wang | Nupur Kumari | Rohan Agarwal | Mia Tang | David Bau | Jun-Yan Zhu |
ACM SIGGRAPH Asia Conference Papers (2023)
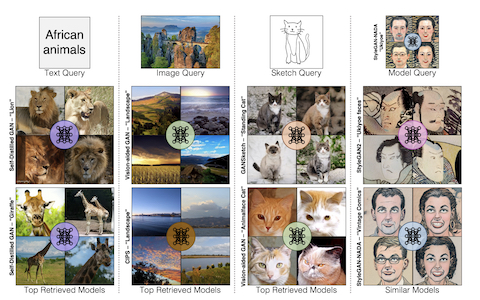
The growing proliferation of customized and pretrained generative models has made it infeasible for a user to be fully cognizant of every model in existence. To address this need, we introduce the task of content-based model search: given a query and a large set of generative models, finding the models that best match the query. As each generative model produces a distribution of images, we formulate the search task as an optimization problem to select the model with the highest probability of generating similar content as the query. We introduce a formulation to approximate this probability given the query from different modalities, e.g., image, sketch, and text. Furthermore, we propose a contrastive learning framework for model retrieval, which learns to adapt features for various query modalities. We demonstrate that our method outperforms several baselines on Generative Model Zoo, a new benchmark we create for the model retrieval task.
Daohan Lu, Sheng-Yu Wang, Nupur Kumari, Rohan Agarwal, Mia Tang, David Bau, Jun-Yan Zhu (2023). Content-based search for deep generative models. ACM SIGGRAPH Asia Conference Papers, 1--12.
@inproceedings{lu2023content,
title={Content-based search for deep generative models},
author={Daohan Lu and Sheng-Yu Wang and Nupur Kumari and Rohan Agarwal and Mia Tang and David Bau and Jun-Yan Zhu},
booktitle={ACM SIGGRAPH Asia Conference Papers},
pages={1--12},
year={2023},
}