Depth-supervised NeRF: Fewer Views and Faster Training for Free
Kangle Deng | Andrew Liu | Jun-Yan Zhu | Deva Ramanan |
Proceedings of the IEEE/CVF Conference on Computer Vision and Pattern Recognition (CVPR) (2022)
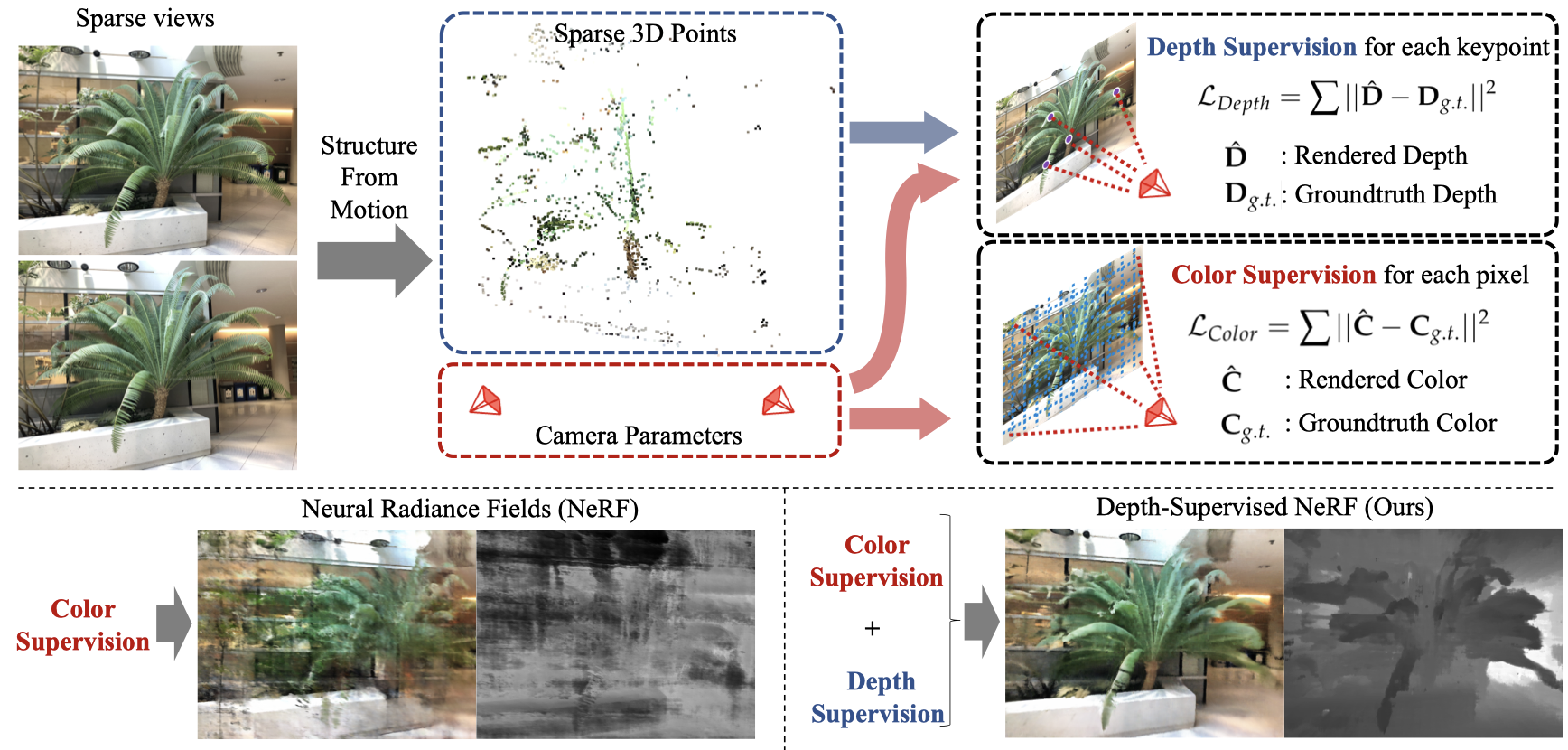
A commonly observed failure mode of Neural Radiance Field (NeRF) is fitting incorrect geometries when given an insufficient number of input views. One potential reason is that standard volumetric rendering does not enforce the constraint that most of a scene's geometry consist of empty space and opaque surfaces. We formalize the above assumption through DS-NeRF (Depth-supervised Neural Radiance Fields), a loss for learning radiance fields that takes advantage of readily-available depth supervision. We leverage the fact that current NeRF pipelines require images with known camera poses that are typically estimated by running structure-from-motion (SFM). Crucially, SFM also produces sparse 3D points that can be used as
Kangle Deng, Andrew Liu, Jun-Yan Zhu, Deva Ramanan (2022). Depth-supervised NeRF: Fewer Views and Faster Training for Free. Proceedings of the IEEE/CVF Conference on Computer Vision and Pattern Recognition (CVPR).
@article{deng2022dsnerf,
title = {Depth-supervised NeRF: Fewer Views and Faster Training for Free},
author = {Kangle Deng and Andrew Liu and Jun-Yan Zhu and Deva Ramanan},
booktitle = {Proceedings of the IEEE/CVF Conference on Computer Vision and Pattern Recognition (CVPR)},
year = {2022},
}