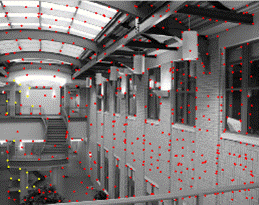
FEATURE
MATCHING for AUTOSTITCHING
Due Date: by 11:59pm, Sun, Nov 13
The goal of this project is to create a system
for automatically stitching images into a mosaic. A secondary goal is to learn how to read and
implement a research paper. The project
will consist of the following steps:
- Detecting corner features in an image
- Extracting a Feature Descriptor for each feature point
- Matching these feature descriptors between two images
- Use a robust method (RANSAC) to compute a homography
- Proceed as in Project 3 to produce a mosaic
For steps 1-3, we will follow the paper “Multi-Image Matching using
Multi-Scale Oriented Patches” by Brown et al. but with several
simplifications. Read the paper first and make sure you understand it. Then implement the algorithm:
- Start with Harris Interest Point Detector (Section 2). We won’t worry about muti-scale – just do a single scale. Also, don’t worry about sub-pixel
accuracy. Re-implementing Harris is
a thankless task – so you can use my sample code: harris.m
- Implement Adaptive Non-Maximal
Suppression (Section 3)
- Implement Feature Descriptor extraction (Section 4). Don’t worry about
rotation-invariance – just extract axis-aligned 8x8 patches. Note that it’s extremely important
to sample these patches from the larger 40x40 window to have a nice big
blurred descriptor. Don’t
forget to bias/gain-normalize the descriptors. Ignore the wavelet
transform section.
- Implement Feature Matching (Section 5). That is, you will need
to find pairs of features that look similar and are thus likely to be good
matches. You may find dist2.m useful for fast distance computations. For thresholding,
use the simpler approach due to Lowe of thresholding
on the ratio between the first and the second nearest neighbors. Consult
Figure 6b in the paper for picking the threshold. Ignore Section 6.
For step 4, use 4-point RANSAC as described in
class to compute a robust homography estimate.
Submit Your Results
You
will need to submit all your code. Show
results of the different steps of your algorithm: the interest points detected,
the various outlier rejection passes, and the final mosaic. Try to apply this automatic method to your
(or your classmates) mosaic images from the previous project.
Bells & Whistles
- (10 points) Add multiscale
processing for corner detection and feature description.
- (10 points) Add rotation invariance to
the descriptors.
- (20 points) Implement panorama
recognition. Given an unordered set
of images, some of which might form panoramas, you need to automatically
discover and stitch these panoramas together. Don’t worry about bundle
adjustment, just see how far you can get with pair-wise homography estimation.