Scaling up GANs for Text-to-Image Synthesis
Minguk Kang | Jun-Yan Zhu | Richard Zhang | Jaesik Park | Eli Shechtman | Sylvain Paris | Taesung Park |
IEEE Conference on Computer Vision and Pattern Recognition (CVPR) (2023)
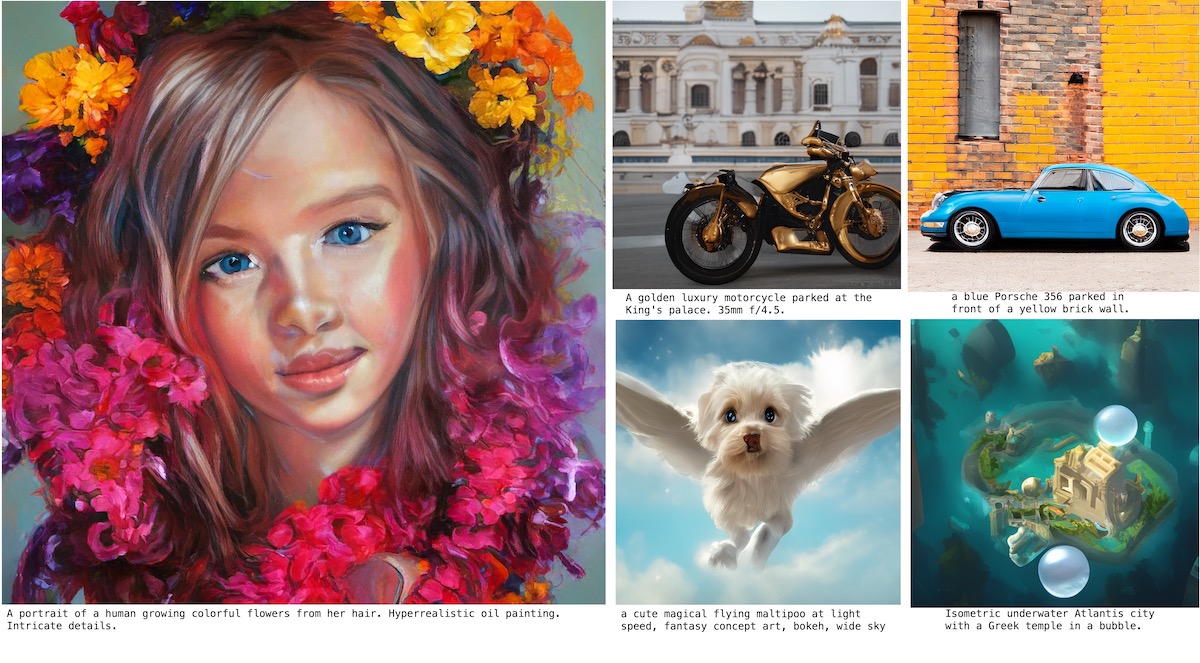
The recent success of text-to-image synthesis has taken the world by storm and captured the general public's imagination. From a technical standpoint, it also marked a drastic change in the favored architecture to design generative image models. GANs used to be the de facto choice, with techniques like StyleGAN. With DALL·E 2, auto-regressive and diffusion models became the new standard for large-scale generative models overnight. This rapid shift raises a fundamental question: can we scale up GANs to benefit from large datasets like LAION? We find that naÏvely increasing the capacity of the StyleGAN architecture quickly becomes unstable. We introduce GigaGAN, a new GAN architecture that far exceeds this limit, demonstrating GANs as a viable option for text-to-image synthesis. GigaGAN offers three major advantages. First, it is orders of magnitude faster at inference time, taking only 0.13 seconds to synthesize a 512px image. Second, it can synthesize high-resolution images, for example, 16-megapixel pixels in 3.66 seconds. Finally, GigaGAN supports various latent space editing applications such as latent interpolation, style mixing, and vector arithmetic operations.
Minguk Kang, Jun-Yan Zhu, Richard Zhang, Jaesik Park, Eli Shechtman, Sylvain Paris, Taesung Park (2023). Scaling up GANs for Text-to-Image Synthesis. IEEE Conference on Computer Vision and Pattern Recognition (CVPR).
@inproceedings{kang2023gigagan,
author = {Minguk Kang, Jun-Yan Zhu, Richard Zhang, Jaesik Park, Eli Shechtman, Sylvain Paris, Taesung Park},
title = {Scaling up GANs for Text-to-Image Synthesis},
booktitle = {IEEE Conference on Computer Vision and Pattern Recognition (CVPR)},
year = {2023},
}