Zero-shot image-to-image translation
Gaurav Parmar | Krishna Kumar Singh | Richard Zhang | Yijun Li | Jingwan Lu | Jun-Yan Zhu |
ACM SIGGRAPH (2023)
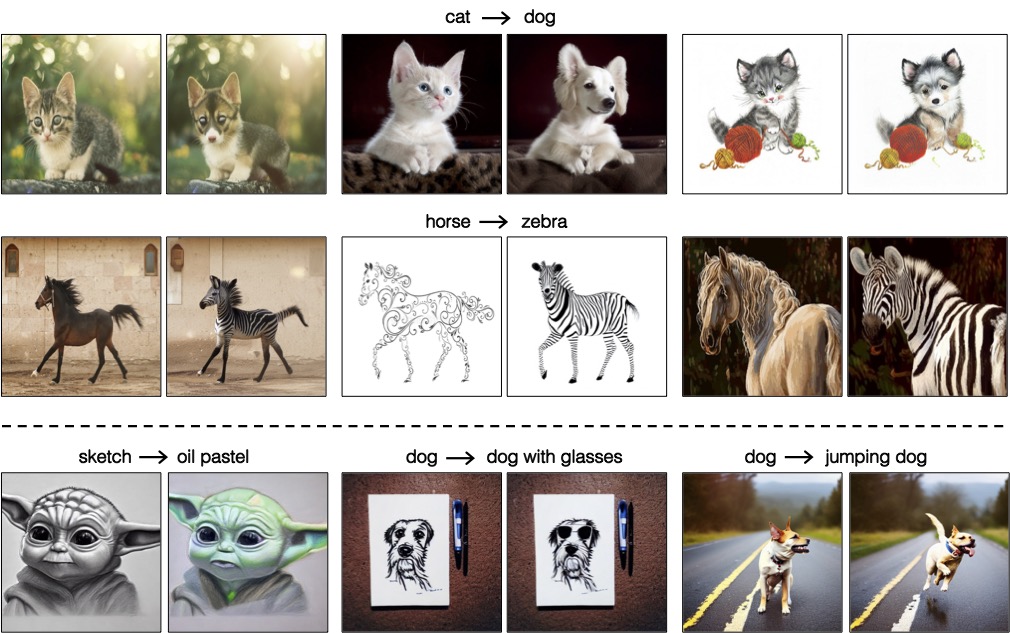
Large-scale text-to-image generative models have shown their remarkable ability to synthesize diverse and high-quality images. However, it is still challenging to directly apply these models for editing real images for two reasons. First, it is hard for users to come up with a perfect text prompt that accurately describes every visual detail in the input image. Second, while existing models can introduce desirable changes in certain regions, they often dramatically alter the input content and introduce unexpected changes in unwanted regions. In this work, we propose pix2pix-zero, an image-to-image translation method that can preserve the content of the original image without manual prompting. We first automatically discover editing directions that reflect desired edits in the text embedding space. To preserve the general content structure after editing, we further propose cross-attention guidance, which aims to retain the cross-attention maps of the input image throughout the diffusion process. In addition, our method does not need additional training for these edits and can directly use the existing pre-trained text-to-image diffusion model. We conduct extensive experiments and show that our method outperforms existing and concurrent works for both real and synthetic image editing.
Gaurav Parmar, Krishna Kumar Singh, Richard Zhang, Yijun Li, Jingwan Lu, Jun-Yan Zhu (2023). Zero-shot image-to-image translation. ACM SIGGRAPH, 1--11.
@inproceedings{parmar2023zero,
title={Zero-shot image-to-image translation},
author={Gaurav Parmar and Krishna Kumar Singh and Richard Zhang and Yijun Li and Jingwan Lu and Jun-Yan Zhu},
booktitle={ACM SIGGRAPH},
pages={1--11},
year={2023},
}